by Kari Lydersen, Energy News Network
An ongoing billing problem in the ComEd area of northern Illinois has some solar companies bracing for turbulence.
When the issue is resolved, community solar subscribers will see a backlog of credits on their utility bills, as well as accrued costs for participating in the project. It’s the kind of surprise that can sour customers on community solar and cause them to opt out of a project.
A new tool being marketed to community solar developers promises to use artificial intelligence to help intervene before such customers churn.
Subscriber churn is known as churn, and it can be a major headache for community solar project owners and managers. Frustration with billing is one of the top reasons people withdraw from projects. Others may leave if they move, or if it takes longer than expected to get a project online.
Churn is “a big problem in the solar community,” says Sam Van Dam, director of asset management for the solar developer 38 degrees north. “It’s something we spend a lot of time on.”
The stakes include lost income and additional uncertainty. In areas where community solar is already popular, it can be challenging to find customers who are still looking for plans. In places where it hasn’t yet been widely embraced, it can mean hours of extra work educating potential subscribers before convincing them to sign up.
Solsticea solar company that works with developers to acquire and serve community solar subscribers is hopeful that artificial intelligence can help companies identify and intervene with customers at risk of churn, as well as community solar more accessible and inclusive in the process.
Solstice has developed an AI tool – now in testing – that predicts when certain subscribers may be vulnerable to churn, based on data from 15,000 accounts on which the AI machine learning model has been trained. This insight allows Solstice to proactively reach out to subscribers who may be in trouble, ensure they feel comfortable with their subscription, and alleviate any concerns or confusion.
If a subscriber is at risk of leaving, advance warning also helps Solstice more efficiently manage the waiting list of prospective subscribers, helping to enroll them more quickly.
Promising figures
Solstice spokesperson Mary Jackson said the AI tool was especially useful when 38 Degrees North transferred management of several thousand subscribers from another company to Solstice, a transition period during which customers may have lost business.
“Using our churn intervention strategies and high-quality customer service, we have retained an astonishingly high number of those subscribers” – 96% of them, Jackson said.
Van Dam called the AI tool ‘a fantastic idea’.
“It becomes particularly challenging when you have hundreds of private customers on one project,” he says. “There’s a lot involved in getting everyone signed up and making sure they continue to pay their bills if they are replaced. If they need to be replaced, this potentially costs money and may lead to loss of turnover. Avoiding that is preferable.”
During a pilot program, Solstice saw churn decrease from 48% to 8.3% among a targeted segment of high-risk customers who they predicted had a greater than 89% chance of churn, the company said. A customer’s length of time as a subscriber and whether a project has consolidated utility billing are important predictors of customer churn.
Solstice data engineer Jake Ford explained that the machine learning tools analyze the training set of data using “advanced algorithms, including deep learning neural networks to detect patterns between variables, gradient boosting classification algorithms and other tree-based models, along with other traditional regression techniques. ”
“All of these models are designed to learn patterns and relationships from large data sets,” he continued. “This dynamic nature of machine learning sets these approaches apart from traditional computer modeling, especially static algorithms that were often hard-coded, meaning little to no flexibility to adapt to new input and data. This is critical, because the inputs or customers we want to analyze in our machine learning applications are often different – locative, demographic, behavioral – than those we trained the models with.”
Redefining risk
Solstice is also hopeful that AI can provide a more accurate and fair way to vet potential solar subscribers. Normally credit scores are used to decide whether someone is likely to pay their bills, but that means people with bad credit due to past financial problems, or those with lower incomes in general, may be left out.
Ford said their AI-based model is known as EnergyScore appears to show that customers who might otherwise be sidelined by a bad credit score are actually a good fit for community solar, as data shows people are likely to pay their utility bills even when finances are tight. This could help those households that need energy savings most access community solar energy.
“When we talk about low-income participation in community solar projects from a developer or financier perspective, their concern comes down to risk: revenue risk, churn risk,” Ford said. “The perception is: the risk is too high, so we don’t include low-income customers. Our data shows that the perception of risk is greater than the actual risk. Not many attempts have been made in the energy sector to measure what the actual risk is.”
Solstice developed EnergyScore “in collaboration with the Department of Energy and data scientists from MIT and Stanford, using data from more than 800,000 individuals across 5,000 variables,” the company says. Testing of the patent-pending product has shown it to be more accurate than FICO scores in predicting solar defaults.
As Solstice uses AI tools to combat marginalization, Ford said they are being vigilant about the known risks of discrimination, racism and other unintended consequences generated by AI.
“It’s about being aware and constantly assessing what your model is doing, and being aware of the impact it has on real individuals on the ground, not just rows in a spreadsheet,” he says. “It is important to be agile and flexible in your methodology.”
Increasing equity
Solstice CEO Steph Speirs said AI tools could be especially useful as community solar grows in popularity and companies strive to equitably manage larger subscriber bases.
“We are at an incredible turning point in the energy transition,” she said. “There has been a focus on the supply side, but there is much more technology that can be applied to the demand side to improve both the customer experience and the perception of projects in the community. We wanted to apply lessons from machine learning to customer behavior and start improving the metrics developers care about most in community solar projects.”
Customer churn data, subscription levels, and collection rates impact a solar developer’s ability to obtain financing for community solar projects.
“If these standards decline, the viability of the project is threatened,” says Speirs, who founded the company in 2016 with the aim to increase the participation of low incomes in community solar energy. “We really need to ensure that these projects have low churn rates and high subscription and collection rates.”
Speirs said currently only about 10% of community solar subscribers are low-income. While that is changing in part thanks to the equity incentives in the Inflation Reduction Act and state solar programs, “as an industry we have work to do.”
“The beauty of these machine learning and AI models is that we can use data to rewrite the historical exclusion that has existed in this sector, and improve the financial viability of these projects so we can do more of them faster building,” Speirs continued. “That helps both sides of the market. It helps developers and financiers build multimillion- and billion-dollar projects, and it helps low-income customers access these projects.”
Solstice isn’t the only company in the space. Erik Molinaro, senior VP of customer experience and operations for solar developer NexampAccording to developers and real estate agents across the board, they are using advanced technology and artificial intelligence to facilitate the recruitment and retention of community solar energy. The company uses AI to create personalized videos that guide customers through the line items on their invoices, he noted.
“Anytime you have something out of the ordinary, it triggers a customer, it’s a pain point,” Molinaro said. “We look at that data and use things like ChatGPT to understand why our customer is calling us, and what we can do to create a better environment.”
Kari Lydersen has been writing for the Energy News Network since January 2011. She is an author and journalist who worked for the Washington Post’s Midwest bureau from 1997 to 2009. article first appeared on Energy News Network and is republished here under a Creative Commons license.
Listen to more in-depth conversations on Solar Builder’s YouTube channel
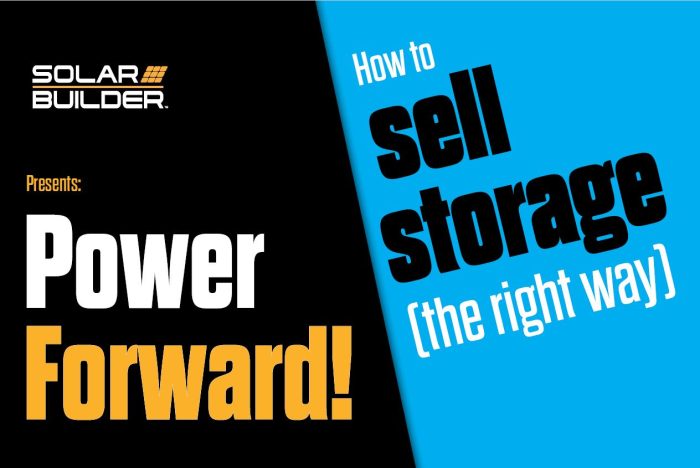
Our most popular series include:
Power forward! | A collaboration with BayWa re to discuss industrial topics at a higher level.
The buzz | Where we give our 2 cents per kWh in the residential solar market.
The pitch | Discussions with solar manufacturers about their new technology and ideas.
Source link