A scientist in Sweden has developed a new hybrid local feature-based method that uses thermographs to identify defective solar panels.
A researcher from Sweden’s Jönköping University has proposed a machine learning-based approach to health monitoring for PV systems using infrared thermography.
The method is based on a hybrid local feature-based approach to monitor panels, designed to resist scaling, noise, rotation and blur. It achieved a training accuracy of 98% and a testing accuracy of 96.8%.
“Existing image processing-based machine learning approaches for health and fault diagnosis are often limited to specific datasets and suffer from issues such as sensitivity to rotation, scaling, noise, blurring and haze,” said Dr. Waqas Ahmed in his article. “These approaches also face trade-offs between memory usage and accuracy. Deep learning-based approaches, while powerful, also require high computational complexity, memory and processing requirements, and are prone to under- and overfitting without strong data sets and careful hyperparameter tuning.”
The new method starts with capturing infrared thermographs with an infrared camera. In the preprocessing step, it assesses the quality of the thermographs and improves it with a dehazing algorithm and contrast adjustment in the grayscale channel if too much noise is present. Each thermographer is then divided into subthermographs of 5×5 pixels.
Local features are extracted from each subthermograph using Gaussian and nonlinear methods. The most important 80% of these attributes are retained, while irrelevant and redundant values are removed. A k-means unsupervised clustering algorithm then reduces the feature vector to 300 elements per thermographer for optimal memory usage.
“Shallow classifiers, such as support vector machine (SVM), train the model on the feature vectors. A fivefold cross-validation approach ensures good model training,” said Ahmed. “A test vector of invisible thermographs is used to test the model’s accuracy in classifying the PV panels into three health-based classes: healthy, hotspot and defective.”
The new method, tested on a 44.24 kW crystalline silicon (c-Si) PV rooftop system in Lahore, Pakistan, consists of eight strings, each with 22 PV modules in series, for a total of 5.28 kW. The system installs 376 PV modules, each with a power of 240 W. Infrared thermographs were recorded at ambient temperatures ranging from 32 C to 40 C, wind speeds of 6.9 m/s and a radiation level of 700 W/m². The thermographs were randomized, with 80% used for training and 20% for validation.
“The results of this study are particularly striking, with the method achieving a remarkable training accuracy of 98% and a testing accuracy of 96.8% with five-fold cross-validation,” said Ahmed. “In addition, the model’s precision values are 92%, 100% and 100%; recall values of 100%, 100% and 90%; and F1 scores of 0.958, 1.0, and 0.947 for the defective, healthy, and hotspot classes, respectively, indicate a high level of performance based on these metrics.”
Compared to other artificial intelligence (AI) approaches in the literature, only the RB scale-invariant feature transformation outperformed the proposed method, with a score of 98.66%. The strongest SURF scored 97.6%, deep neural features (pre-trained network) and shallow classifier scored 97%, RGB, LBP, average HOG and texture scored 96.8%, isolated CNN scored 96%, and Texture, HOG and PCA scored 94.1%.
Ahmed presented the results in “Improving solar PV reliability with hybrid local functions and infrared thermography”, which was recently published in Energy reports.
This content is copyrighted and may not be reused. If you would like to collaborate with us and reuse some of our content, please contact: editors@pv-magazine.com.
Popular content
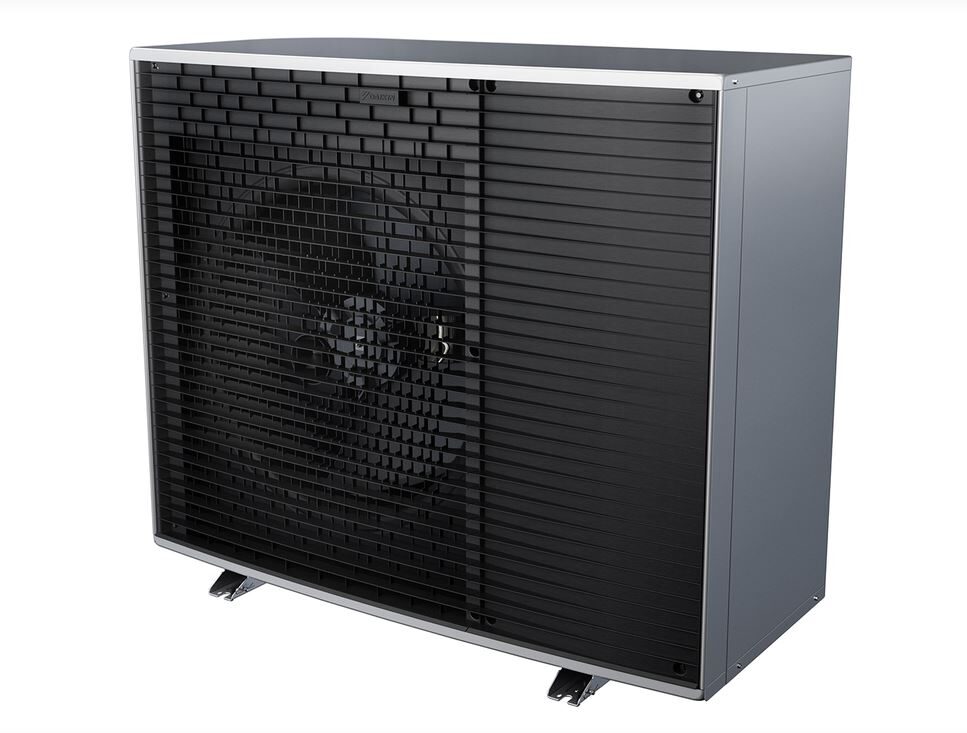